Home
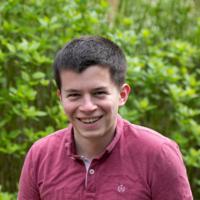
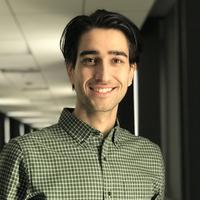
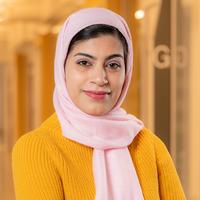
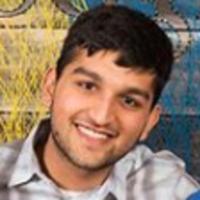
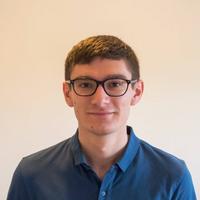
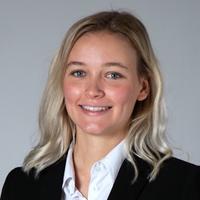
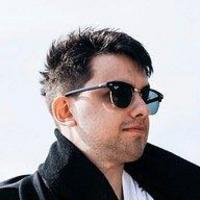
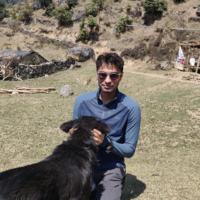
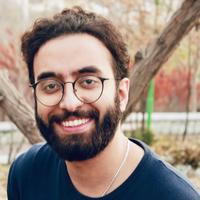
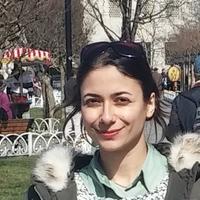
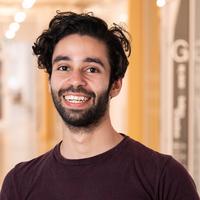
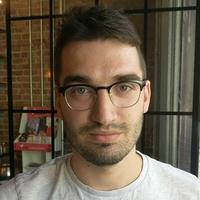
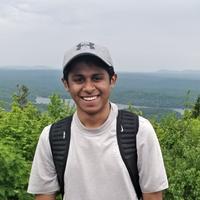
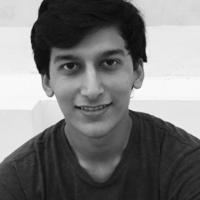
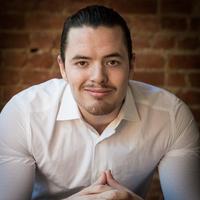
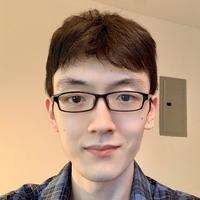
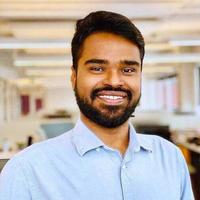
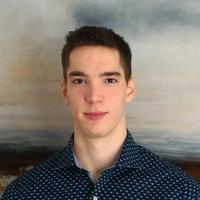
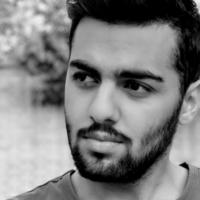
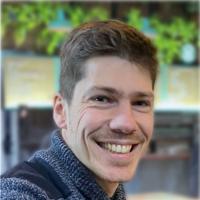
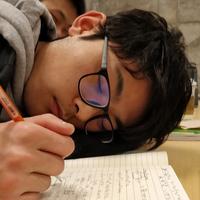
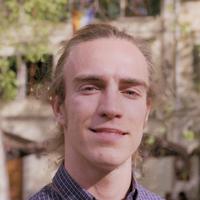
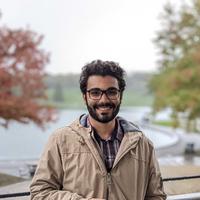
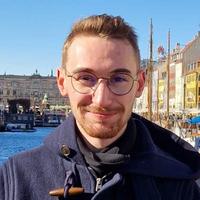
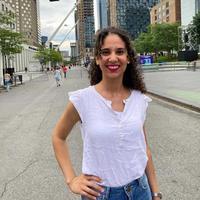
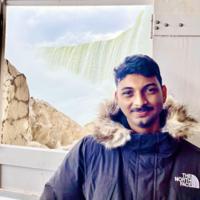
About the Lab
Chandar Research Lab (CRL) is a machine learning research group in the Department of Computer and Software Engineering at Polytechnique Montreal. The long-term mission of our group is to develop interactive learning algorithms that continuously learn from experiences. Currently, the lab focuses on several areas in machine learning including Deep Learning, Reinforcement Learning, Lifelong Learning, and Natural Language Processing.
CRL is also affiliated with Mila, the Quebec AI Institute.
News
-
(December 2024) One work on building LLMs for 3D molecule generation accepted to AAAI 2025.
-
(October 2024) One work on context length in reinforcement learning accepted to NeurIPS.
-
(September 2024) Two papers on knowledge understanding in LLMs and speculative decoding accepted to EMNLP and another on LLM quantization accepted to Findings.
-
(August 2024) One work on attention modulation for fairness accepted to COLM.
-
(May 2024) Our work on self-explanations from large language models was accepted at Findings of ACL 2024.
-
(May 2024) Two papers accepted at ACL 2024: prompt-based fairness metrics correlation and parameter-efficient preference alignment.
-
(May 2024) Two papers accepted at ICML 2024: faithfulness measurable masked language models and Lookbehind-SAM.
-
(April 2024) Our work on sub-goal distillation was accepted at CoLLAs 2024.
-
(January 2024) One work on multi-agent Hanabi accepted to 2025.
-
(January 2024) Two papers accepted at ICLR 2024: intelligent switching in reset-free RL and mastering memory tasks with world models.
-
(January 2024) Our work on offline reinforcement learning for crystal design was accepted at Digital Discovery.
-
(January 2024) Our work on fairness-aware pruning in Transformers was accepted at AAAI 2024.
-
(October 2023) Three papers accepted at EMNLP 2023: knowledge acquisition-utilization gap in LLMs, evaluation of LLMs, and data reweighting for LLMs.
-
(May 2023) Our work on cooperative multi-agent reinforcement learning was accepted at UAI 2023.
-
(May 2023) Two papers accepted at CoLLAs 2023: adaptive model-based reinforcement learning and few-shot coordination in Hanabi.